How can a retailer identify the products that keep customers coming back? In collaboration with Coop Eesti, STACC developed a data-driven solution that offers customers personalized product discounts based on their preferences. Leveraging machine learning, we designed an innovative model that personalizes the shopping experience and strengthens customer loyalty. This solution is unique in Estonia, as the personalized discounts are not displayed on price tags but tailored specifically for each customer.
Opportunities for data-driven solutions at Coop Eesti
Coop Eesti, a leader in the Estonian retail market, has over 120 years of history. Holding nearly 24% of the market share, the company boasts a net revenue of €863 million and operates 320 stores across the country. Over 600,000 loyalty cardholders – roughly equivalent to the number of working people in Estonia – frequent Coop Eesti stores regularly, alongside hundreds of thousands of other customers without loyalty cards. This massive pool of data offers unparalleled opportunities for creating data-driven solutions and delivering a more personalized shopping experience – a vision that Coop has successfully realized with STACC’s help.
Personalized pricing program: what is it and how does it work?
Coop’s personalized pricing program is an innovative customer-centric solution that allows shoppers to benefit from discounts tailored to their individual preferences and buying habits. This approach goes beyond traditional promotional offers, where all customers receive the same discounts regardless of their shopping behavior or needs. Coop’s pilot project in Estonia demonstrates how personalization can make the shopping experience more flexible and engaging.
The system, powered by artificial intelligence, analyzes customers’ past purchasing behavior to select six products that align with their preferences. These can be confirmed by the customer as permanent personalized offers. If a product becomes less relevant, customers can easily update their selection in the client portal or app. Additionally, the algorithm suggests three more products from Coop’s range that the customer might enjoy trying, ensuring even the most discerning shoppers find discounts on their favorite items.
To keep offers relevant, personalized discounts are updated monthly, reflecting changes in customer habits. The customer’s confirmed favorite products remain unchanged, with discounts applied as long as the product is in stock or until the customer decides to make a switch. Similarly, the list of alternative recommendations is refreshed regularly to ensure flexibility and choice. This dynamic process keeps the customer at the heart of the shopping experience.
Here’s an example of personalized offers that have been confirmed as permanent discounts for a Coop loyalty cardholder who works at STACC:
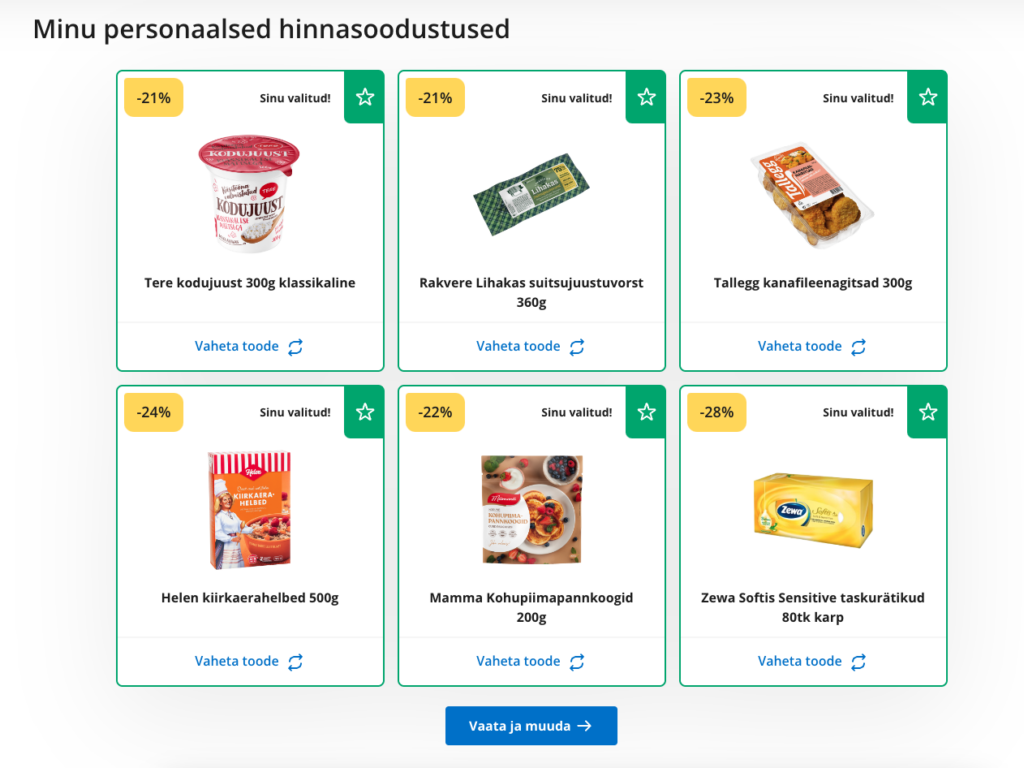
It’s a colleague who enjoys quick and easy meal preparation and is pleased that the selected products make their typical grocery purchases more affordable.
Innovation that bridges customers and technology
While other retailers also offer personalized recommendations among promotional products, Coop takes personalization to the next level by making customers active participants in shaping their shopping experience.
What makes this solution unique?
- Individualized discounts: Personalized offers are available exclusively to loyalty cardholders who meet the conditions for receiving them, ensuring discounts are tailored to their preferences.
- Machine learning and customer collaboration: The machine learning model analyzes customer data to generate new recommendations every month. However, automation isn’t the only focus – customers can actively shape their offers by confirming or adjusting recommendations via the Coop app or portal. This creates a dynamic, personalized interaction between the model and the customer.
- Continuous and evolving process: Once customers confirm their selected products, discounts remain valid until the product is unavailable or the customer chooses to replace it. If no selection is made, the system suggests new products each month, offering fresh and relevant alternatives.
The principles behind the machine learning model
Coop’s personalized pricing model is based on customers’ purchase history. It segments this data to identify both stable purchasing habits and recent behavioral changes. Seasonal trends are also factored in, ensuring, for instance, that winter months bring discounts on items like blood sausages and mulled wine. Additional customer details, such as age and gender, further refine recommendations, making them even more accurate and relevant.
The model doesn’t rely solely on an individual’s data but also considers purchasing patterns of similar customers. For example, if two customers frequently buy the same products, the system can recommend items that one has enjoyed but the other hasn’t yet discovered. This opens the door to new favorites that customers may not have tried otherwise. The model continually improves itself, learning from past recommendations to deliver more precise and relevant offers. If a previous suggestion failed to resonate, the system ensures it doesn’t repeat the same mistake.
Rather than a big “black box” where data is input, and results are output, this solution is a complex combination of several machine learning models working together at different stages. The philosophy behind its creation ensures that each step compensates for any gaps in the previous ones, delivering optimal results for every customer. Feedback – analyzed through purchasing behavior – shows that the recommendations resonate with customers while also generating economic benefits for Coop.
Impact on the customer experience
Personalized pricing reflects a broader retail trend of prioritizing individual customer needs and preferences. This approach helps retailers increase customer loyalty by adding value and showing they truly understand their shoppers. It also allows customers to optimize their spending on items they care about most, providing reassurance about future discounts – something traditional promotions often lack.
Coop’s example demonstrates that personalized pricing isn’t just a future trend; it’s a current reality that makes shopping smarter and more customer-friendly. The solution developed in collaboration with STACC highlights how data-driven innovation can create a truly personalized shopping experience.
If you’re interested in implementing data-driven solutions for your business, contact us to discuss how we can help you build innovative solutions that give your company a competitive edge.