From a socio-economics perspective, rural and agricultural life has brought challenges and opportunities. One of the challenges is predicting yields: how do you know when and how much yields will come, and how do you optimize them? The answer may lie in a recent project carried out by STACC and eAgronom.
Predicting yield
eAgronom is a company developing agricultural software that, in collaboration with STACC, developed an intelligent artificial vision system that allows predicting the yield of winter wheat fields this season based on satellite imagery. The Sentinel satellites launched under the Copernicus program of the European Union enable near real-time land monitoring while ensuring free access to the data. Knowledge based on satellite imagery is at the forefront of contemporary agricultural research and development, with initiatives like eAgronom’s crop yield prediction project taking advantage of these insights. The application created in the project is able to read the multi-spectral data of satellite images, process them as a time series (Figure 1), and evaluate the crop yield of winter wheat fields with an artificial neural network.
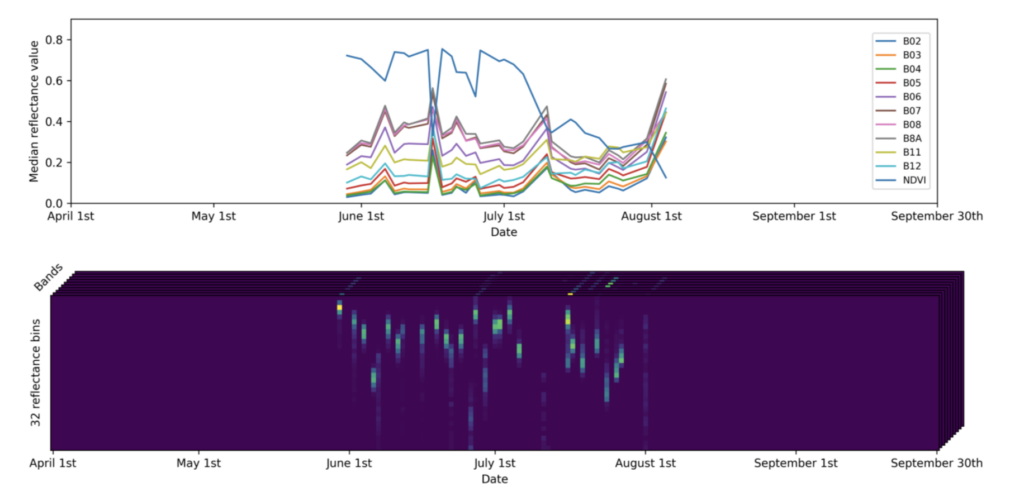
Figure 1. Example time series of a field. Two aggregation approaches were tested as model input: median and histogram
Effective model
The project compared classical machine learning algorithms as well as modern artificial neural network architectures as potential yield prediction models. Still, particular attention had to be paid to the accuracy metric. Typically, root-mean-square error (RMSE) is used as an evaluation metric in similar regression tasks. However, when predicting crop yield, the range of possible yield values between two studies may vastly differ. For example, average Polish winter wheat yield may be much higher than that of Estonia’s. Therefore, the RMSE metrics between the two studies, which focus on the same crop in different regions, are not directly comparable. So in order to ensure the comparativeness of the project with previous scientific literature, the RMSEs in all experiments were normalized through division by average ground truth yield. Quite indicatively, this metric is called the normalized root-mean-square error (NRMSE).
“One of the most prominent results of the project is the ability to predict yields as early as six weeks in advance, with similar accuracy as just before the harvest. It can also be confirmed that the model created in the project is equivalent to the latest scientific literature results.”
— Ingvar Baranin, data scientist involved in the project
The best result from the solutions tested was a neural network based on LSTM neurons, which was able to model yields with an NRMSE error of 0.25. Researchers from Finland and Australia have obtained similar results in recent scientific literature [1, 2].
Contribution to the development of agriculture
The collaborative project between eAgronom and STACC has ushered in notable advancements in Estonian agriculture. By harnessing satellite imagery combined with artificial neural networks, a model was developed that forecasts crop yield well in advance of the harvest season. This pivotal innovation aids farmers in optimizing resource allocation and enhancing future planning.
“Forecasting yields as accurately as possible will help the farmer to plan harvesting, the logistics of transporting crops, the need for storage space, the process of drying crops, the sale of crops, and post-harvest operations in the field, such as spreading the head and cultivating the land.”
— eAgronom’s agronomist Piibe Vaher
If your company needs a similar study, STACC is ready to help you!
Sources:
1. Fiona H. Evans and Jianxiu Shen. Long-Term Hindcasts of Wheat Yield in Fields Using Remotely Sensed Phenology, Climate Data and Machine Learning. Remote Sensing, 13(13), 2021.
2. Maria Yli-Heikkila, Samantha Wittke, Markku Luotamo, Eetu Puttonen, Mika Sulkava, Petri Pellikka, Janne Heiskanen, and Arto Klami. Scalable Crop Yield Prediction with Sentinel-2 Time Series and Temporal Convolutional Network. Remote Sensing, 14(17), 2022.