Did you know that artificial intelligence can also be used in museums? The Estonian National Heritage Board launched an exciting project called kratt Sälli* whose purpose is to make conducting inventories in museums quicker and more convenient. Due to the large amount of museum objects, it is not feasible for museum staff to thoroughly examine them all. STACC and its top-level data scientists came to help the Estonian National Heritage Board to create an artificial intelligence solution for the preservation of museum objects.
* Kratt is a magical creature in old Estonian mythology built from hay or old household items to serve its master. In Estonia, kratt is used as a metaphor for AI. Sälli comes from “säilitamine”, the Estonian word for preservation.
What is Sälli?
Sälli is a decision support system that predicts the stability of museum objects and helps to draw the attention of museum staff to objects whose condition checks should be prioritized due to their physical properties or frequent use in order to plan activities that could prevent condition deterioration. Sälli could help museums to implement a flexible inventory process where instead of conducting inventories with fixed intervals, objects are checked when necessary.
The results of the Sälli project are highlighted in the December 2023 issue of the International Journal of Conservation Science. For more detailed information about the technical work and machine learning models of the Sälli project, please refer to the following link: https://ijcs.ro/volume-14-2023/#Issue4
What problem did the National Heritage Board need STACC to solve?
According to the Estonian National Heritage Board, the inventory of a single museum object by two people takes 19 minutes on average. The Museums Act states that museums must conduct inventories such that each museum object would be checked at least once every five years. Approximately 2,5 million objects from over 20 museums are planned to be moved to two common repositories after their completion in 2027. In theory, conducting inventories for all of them takes 90 years of work hours, which, spread out over five years, means that over 70 people should be hired full time just for inventories.
In an ideal world, in addition to checking the presence and location of a museum object, the inventory process also includes reviewing the object’s condition and defects as well as updating and correcting the information about it in the museums’ information system. Due to insufficient staff, the large quantity of museum objects, and slow, unoptimized processes, these additional activities often remain undone and it is not feasible to monitor the condition and long-term stability of objects or to plan activities that could prevent condition deterioration.
“STACC tried to solve the problem of time-consuming inventory and stability evaluation. The goal wasn´t to find a working solution right away, for us it was important to analyze the data in MuIS and then experiment with potential models. STACC managed it very well. The analysis was comprehensive and both the museums and the National Heritage Board have a lot to learn from the conclusions.”
Janika Turu, Artistic Heritage Adviser of the Department of Museums and Artistic Heritage in the National Heritage Board and member of Sälli steering group
With the common repositories already under construction, it is appropriate and necessary to explore options for optimizing and simplifying the steps and activities in the inventory process. In order to reduce the time spent on inventories, it is necessary to start from the bottlenecks of the process. Evaluating an object’s condition and defects and estimating its lifetime adequately requires the knowledge of conservationists or preservation specialists, and time to carefully unpack and examine the object, but due to a shortage of time, this is often not possible. Furthermore, some museums do not have their own specialists with the necessary knowledge. Therefore, it would be helpful if artificial intelligence could be used to predict the stability of an object’s condition based on its properties and storage conditions. STACC’s main task was to assess whether it is possible to create an artificial intelligence solution for predicting the stability of an object and whether it would alleviate the problems related to the inventory process.
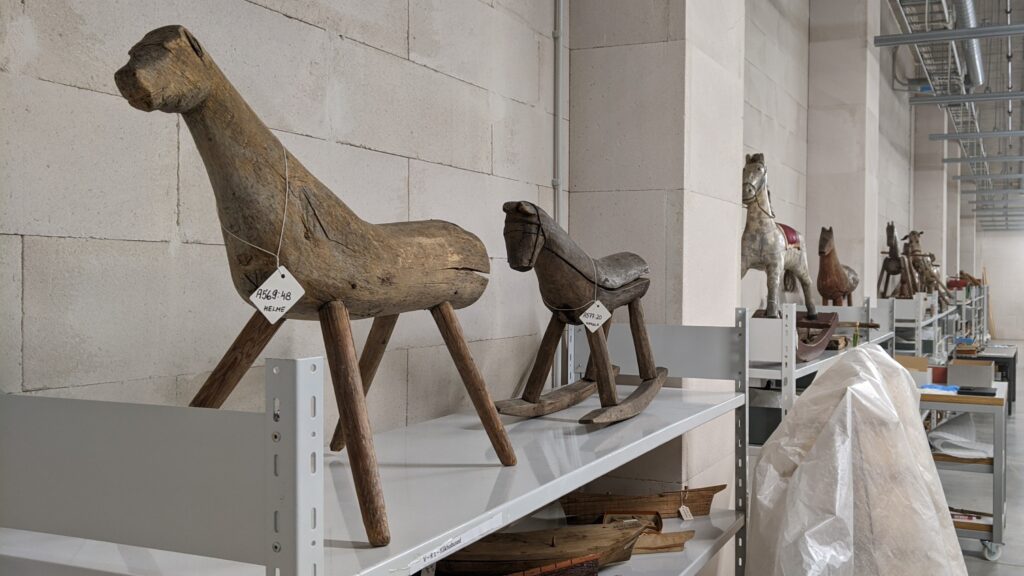
How did STACC solve this problem?
STACC obtained the data required for this task from the museums’ information system MuIS. “The whole analysis and modeling are based on objects’ historical condition evaluations, which could be used to detect when the condition got worse. Most of the objects did not have a long enough history of condition evaluations to model how the condition changes in time. However, it is not necessary to know in what exact condition an object is at a given point in time. Instead, it is important to know whether the condition is stable or there is a chance that it will get worse in the near future. If an object is in a bad but stable condition, it does not need attention as quickly as an object in good condition with a higher probability of getting worse,” explained Meri Liis Treimann, data scientist at STACC.
Considering the available data, the speed of processes in materials, and the fact that museums make long-term work plans years in advance, STACC decided to create a machine learning model that predicts whether the condition of an object will get worse within the next 10 years. We broke the historical time series down to single conditions to which we added labels indicating whether the condition got worse within the following 10 years or not. In addition to conditions, descriptive features of the objects, such as material and date, and events related to the objects, such as exhibitions and conservations, were used for model training.
What were the results?
“We experimented mainly with different tree-based machine learning methods like decision tree, random forest, and gradient boosting,” said Treimann.
For the National Heritage Board, it was important that the model could detect as many unstable objects as possible, which is why the most important model performance metric was recall – the share of worsening objects that the model identified correctly. However, recall alone is not enough – a model that always predicts that an object will deteriorate has a recall of 100%, but such a model would not be useful in practice. To tackle this problem, we used precision as a complementary metric that shows how many of the objects predicted to get worse actually got worse – the higher the precision, the fewer false positives.
Both of the metrics depend on the choice of decision threshold – the minimum probability at which we say that the condition will deteriorate. By choosing a lower threshold we might achieve a higher recall but lose in precision. Every object marked as potentially unstable by the model means that museum staff should go and check its condition, so we cannot lower the decision threshold too much – otherwise there would be too many false positives and the model would not serve its purpose. We agreed that for comparing the models, precision can be as low as 50%, which means that up to twice as many objects can be checked in order to find the unstable objects.
STACC achieved the best results with a random forest model that could detect 92% of worsening objects at 50% precision. This means that, in theory, 184 objects should be checked in order to find 92 out of 100 worsening objects.
How will Sälli be used in the future?
The analysis and solution developed during the pilot project is mainly an important input for developing a production-ready solution in the future. The preliminary analysis and experiments showed that there is potential in the existing data to predict condition deterioration. However, the data need some improvements and the model trained on them is not fully ready and trustworthy from the users’ perspective to undergo a complete transition from fixed-interval inventories to needs-based inventories.
“In my opinion, the knowledge gained from the conclusions of the analysis and results of the experiments brought the greatest benefit. Thanks to the prototype testing we discovered shortcomings in MuIS data and understood which gaps we should fill in data quality and people’s knowledge. We hope to move on with further developments once these gaps are filled,” said Turu.
Museum data have not been collected with the aim of applying machine learning and the analysis brought out some of the shortcomings in data quality. The results of the analysis could be used to improve the data model of MuIS 2.0 as well as museum staff training regarding data entry.
Summary
Artificial intelligence can also be efficiently used in traditional organizations. Even though the data which are not collected with the aim of applying machine learning or data analysis might not be complete or precise, we could still find useful patterns and improve organization processes and data collection, given enough data.
“Collaboration with STACC was very smooth and constructive. Project management from STACC’s side was strong and consistent, which led to a quick growth in trust. We knew that the project was in competent and good hands. STACC is a very reliable partner and the collaboration was pleasant in every way. We hope to meet again in future projects,” said Turu.
Keywords: National Heritage Board, preservation of cultural heritage, museum, random forest.
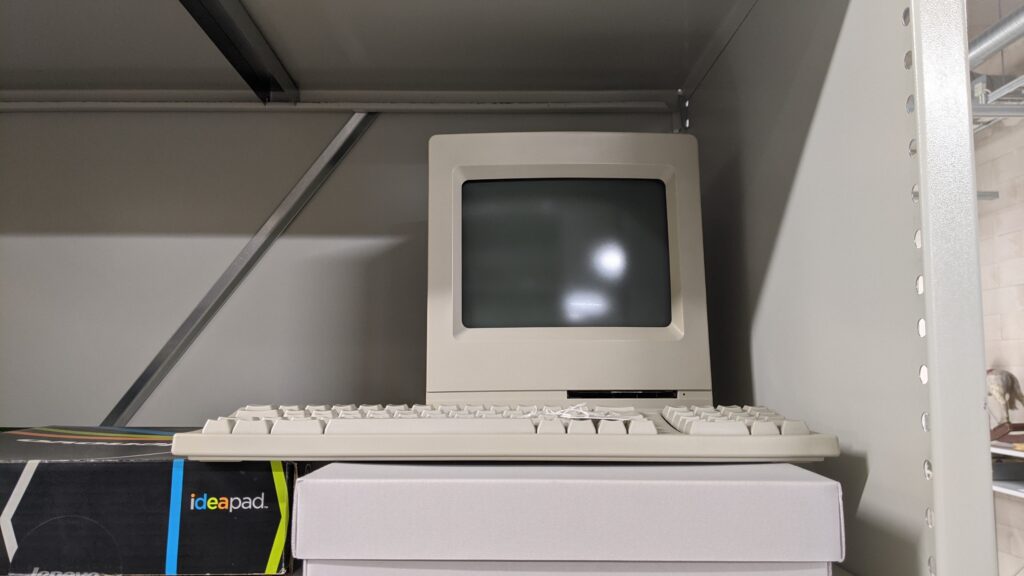
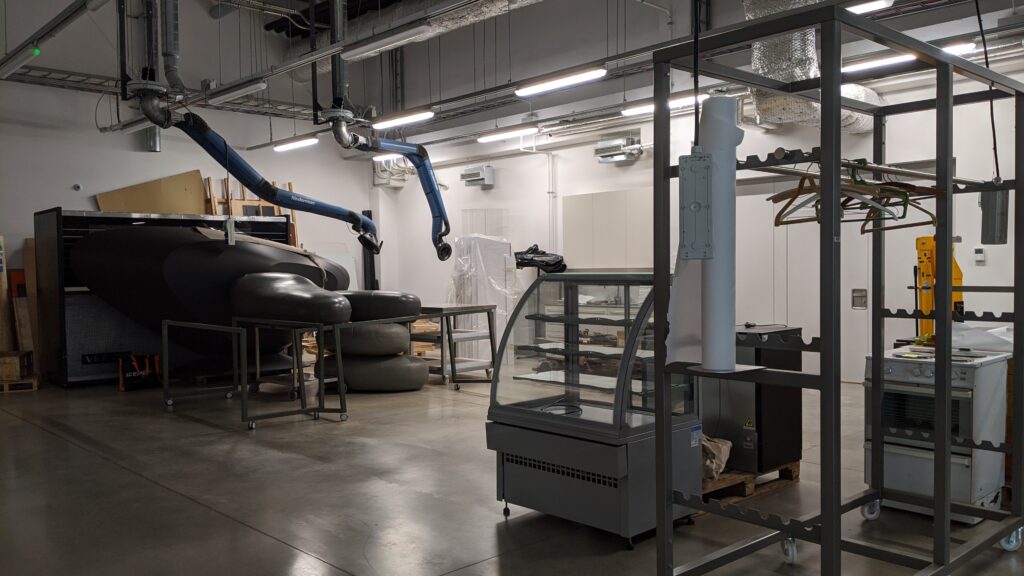